Hi all,
For those that were interested in the discussion about short term trading strategies, holding for only a couple of days etc.
I thought I'd just post an update on the (basic) RSI system that I currently trade on MSFT, its the same one I used in the example.
*** The biggest drawdown is always just around the proverbial corner *** but this last month has finally seen new equity highs in the sytem.
Moving forward if people are interested, I'll post the results of this system whilst I'm still trading it. I'll also show, if / when I stop.
Cheers
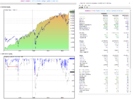
For those that were interested in the discussion about short term trading strategies, holding for only a couple of days etc.
I thought I'd just post an update on the (basic) RSI system that I currently trade on MSFT, its the same one I used in the example.
*** The biggest drawdown is always just around the proverbial corner *** but this last month has finally seen new equity highs in the sytem.
Moving forward if people are interested, I'll post the results of this system whilst I'm still trading it. I'll also show, if / when I stop.
Cheers
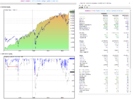